New Research by Dr. Abolfazl Karimpour and Peers Provides Framework For Transportation Agencies to Improve Ramp Control Strategies
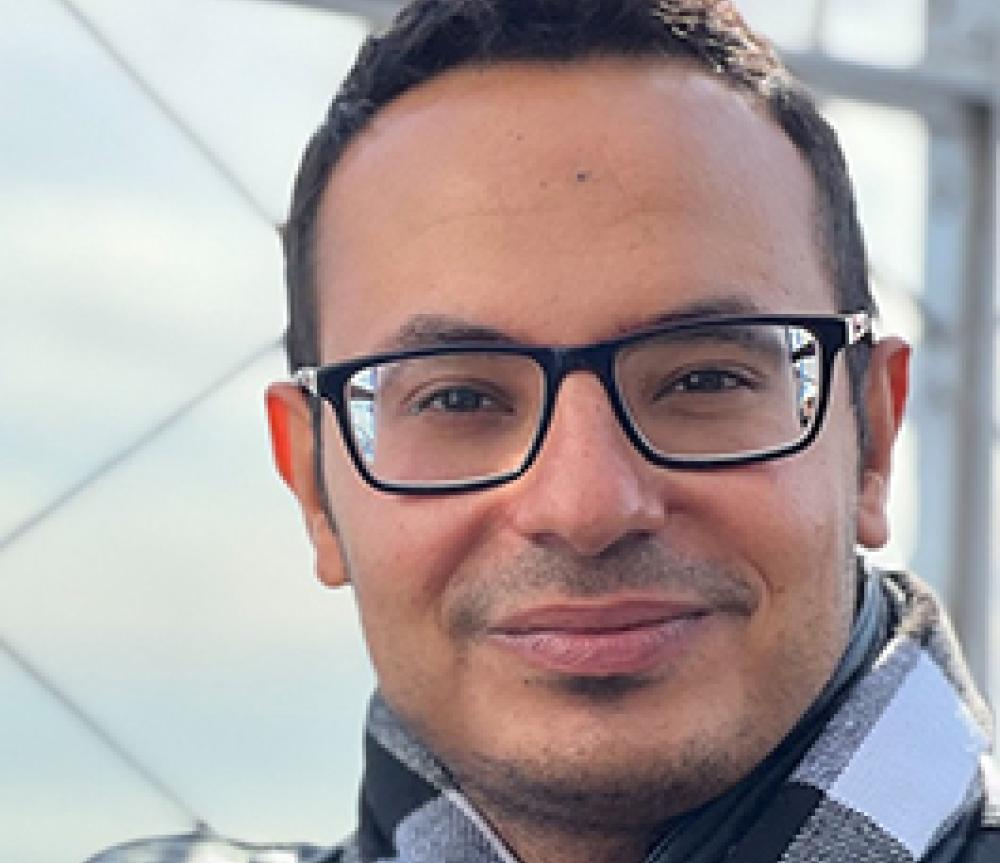
SUNY Polytechnic Institute Assistant Professor of Transportation Engineering, Dr. Abolfazl Karimpour, and peers from the University of Arizona have published new research in the Journal of Intelligent Transportation Systems titled, “Data-driven transfer learning framework for estimating on-ramp and off-ramp traffic flows.” The research is significant because it introduces a data-driven framework using transfer learning to estimate freeway ramp flows accurately from mainline loop detector data.
To develop the most appropriate control strategy and monitor, maintain, and evaluate the traffic performance of the freeway weaving areas, Departments of Transportation need access to traffic flows at each pair of on-ramp and off-ramp, Karimpour explains. However, ramp flows are not always available to transportation agencies. The estimated ramp flows based on the newly proposed framework by Karimpour and peers, can help transportation agencies enhance the operations of their ramp control strategies for locations where physical sensors are not installed.